What is Ground Truth?
A self-driving vehicle is a complex technology built on a simple principle: The world it perceives must match the world as it really is. For creators of self-driving technology, the only way to know if the virtual and real worlds are in sync is through manually verifying that the virtual world aligns with human-defined measurements of accuracy — using what engineers refer to as the “ground truth.”
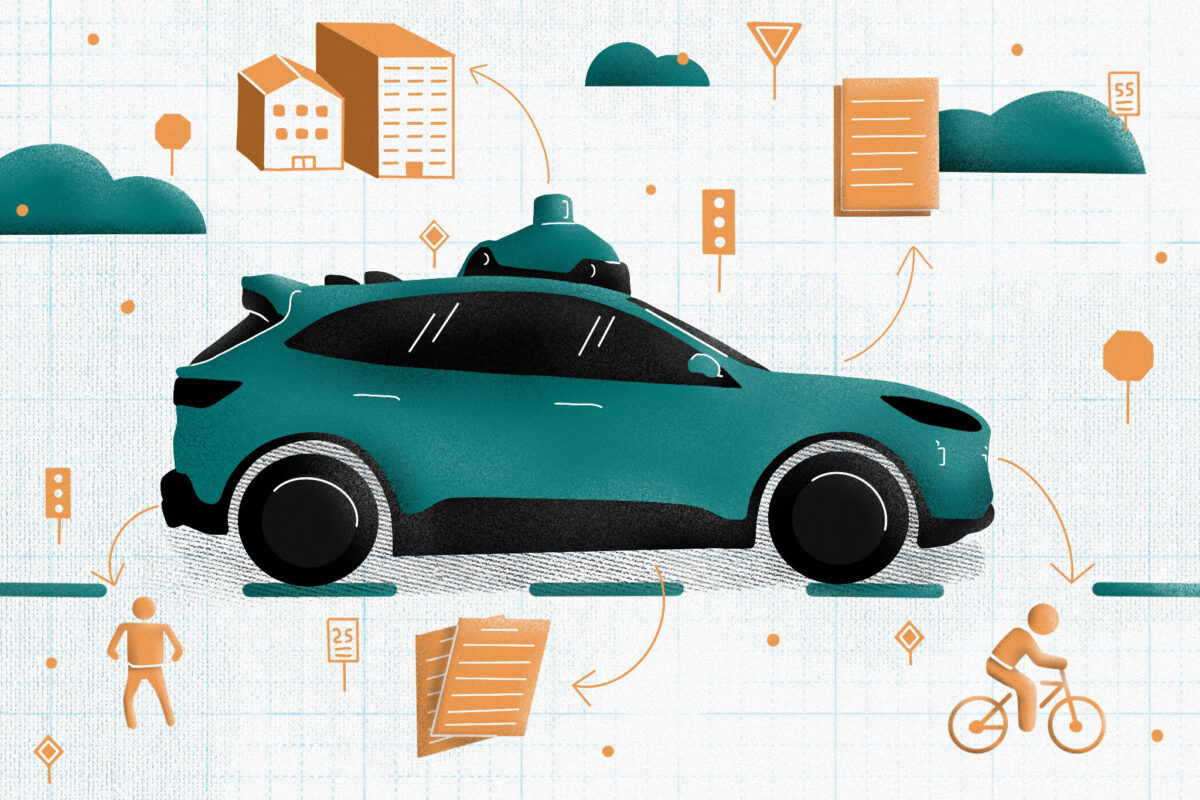
In the context of a self-driving system, ground-truth data is most commonly used to train and validate the machine learning algorithms that comprise what is thought of as the “brain” of a self-driving vehicle. Ground truth can be obtained by a team of expert human annotators when the information processed by a self-driving vehicle’s sensors is consistent with what the naked eye sees. It’s the gold standard of verifying accuracy.